ExRBM
Automatic Fault Diagnosis and
Predictive Maintenance Solution for Machinery
ExRBM analyzes real-time data collected through sensors,
applying AI algorithms based on fault mechanism big data and expert knowledge of machinery, to automatically diagnose machinery faults with over 98% accuracy,
providing the root cause and countermeasures.
applying AI algorithms based on fault mechanism big data and expert knowledge of machinery, to automatically diagnose machinery faults with over 98% accuracy,
providing the root cause and countermeasures.
Why ExRBM?
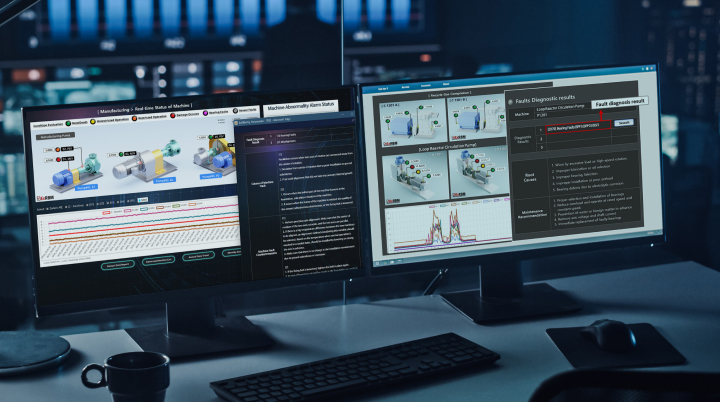
Intuitively Designed Interface for Easy and Accurate Operation
- Real-time monitoring of precise machinery status by location through 3D images and four-level alarms
- Even non-experts can easily understand the machinery's status intuitively
Highly Reliable Data Extraction through Precision Automatic Filtering Technology
- Selective filtration of irrelevant data for reliable data extraction
- Automatic analysis feature enables quick analysis of more data in less time
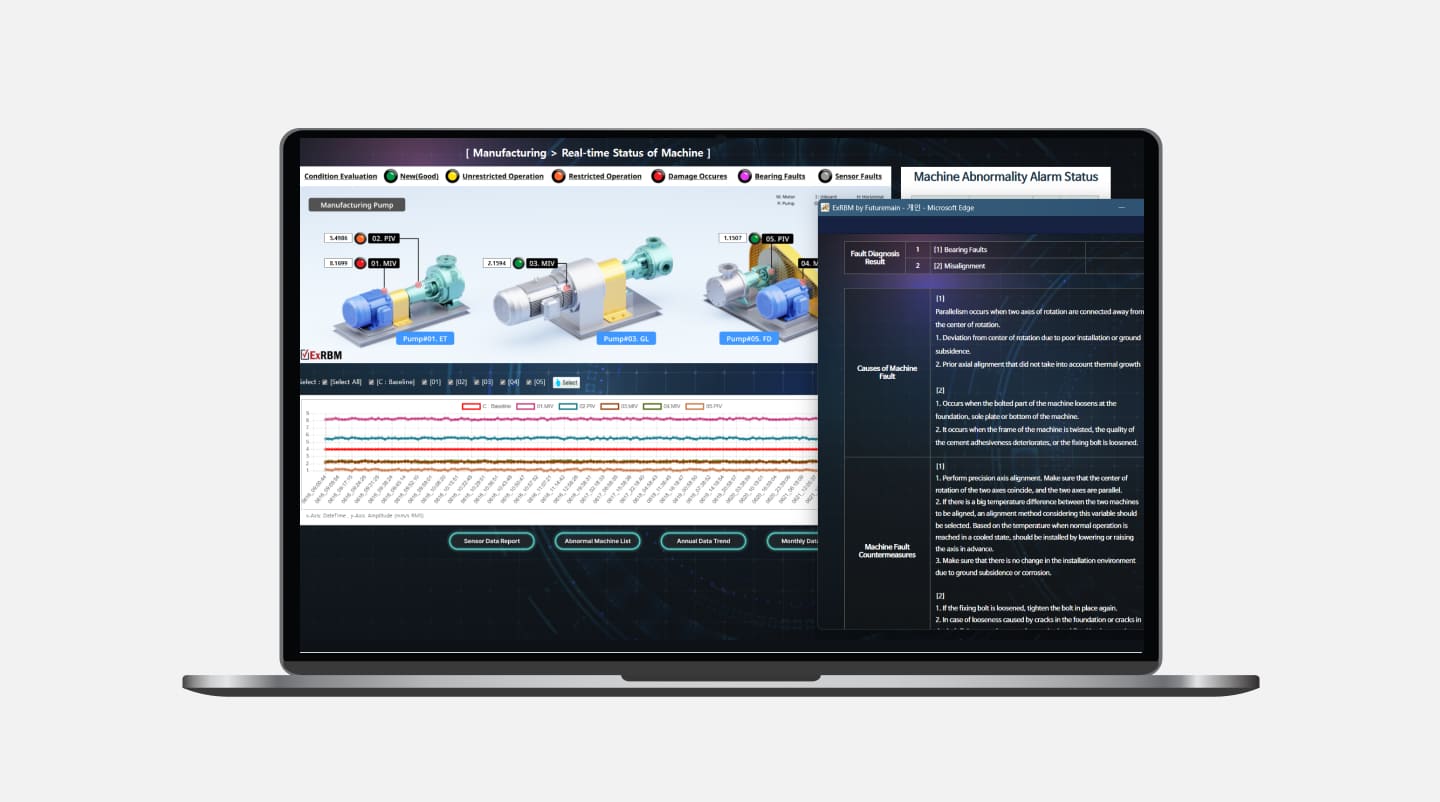
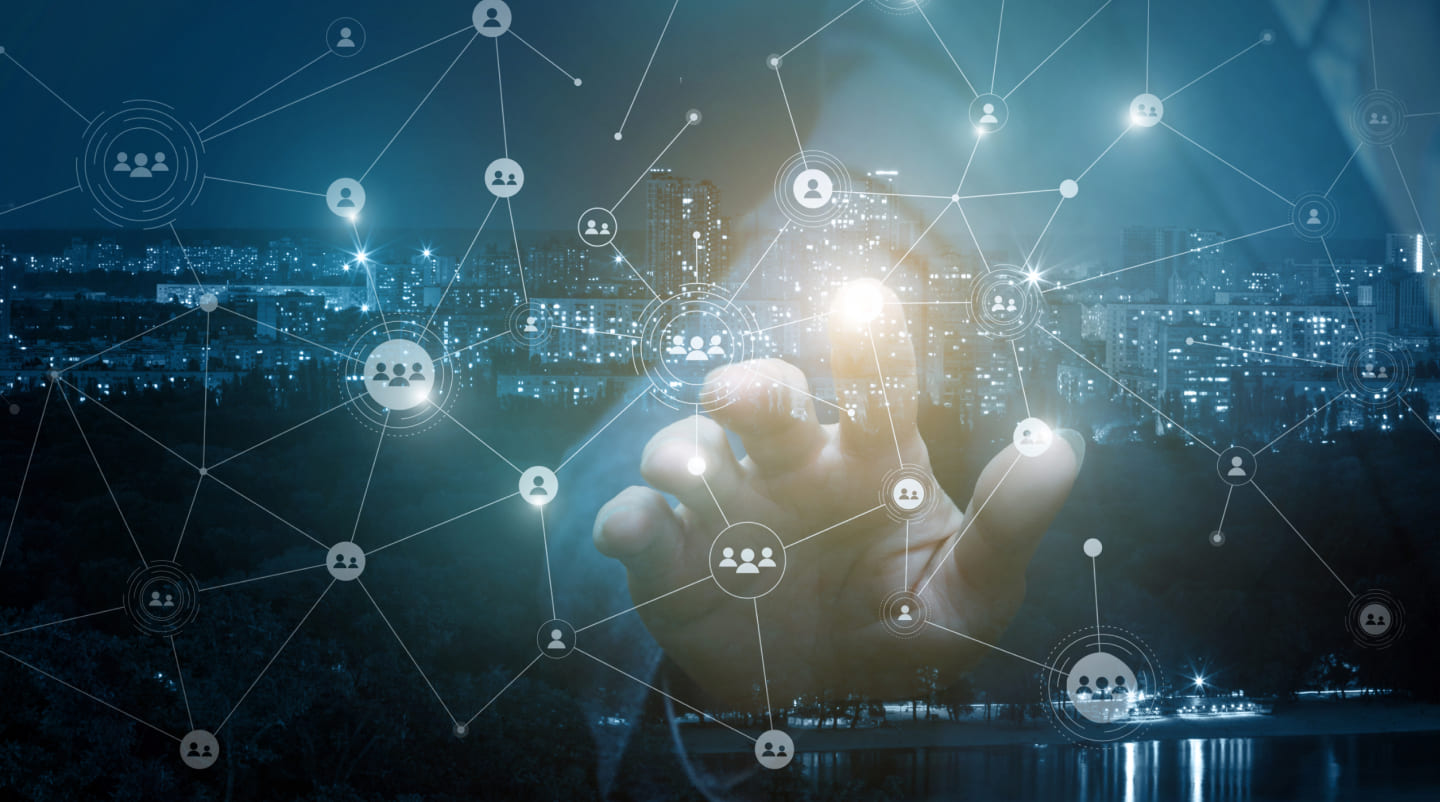
Easily Perform Automatic Diagnosis with just Single Click
- With just single click, view diagnosis results, fault causes, and maintenance countermeasures all at a glance
Rational Construction is Achievable with High Compatibility
- Compatible with existing VMS systems, enabling cost-effective implementation
- Compatible with various sensors such as vibration, temperature, current, voltage, and humidity
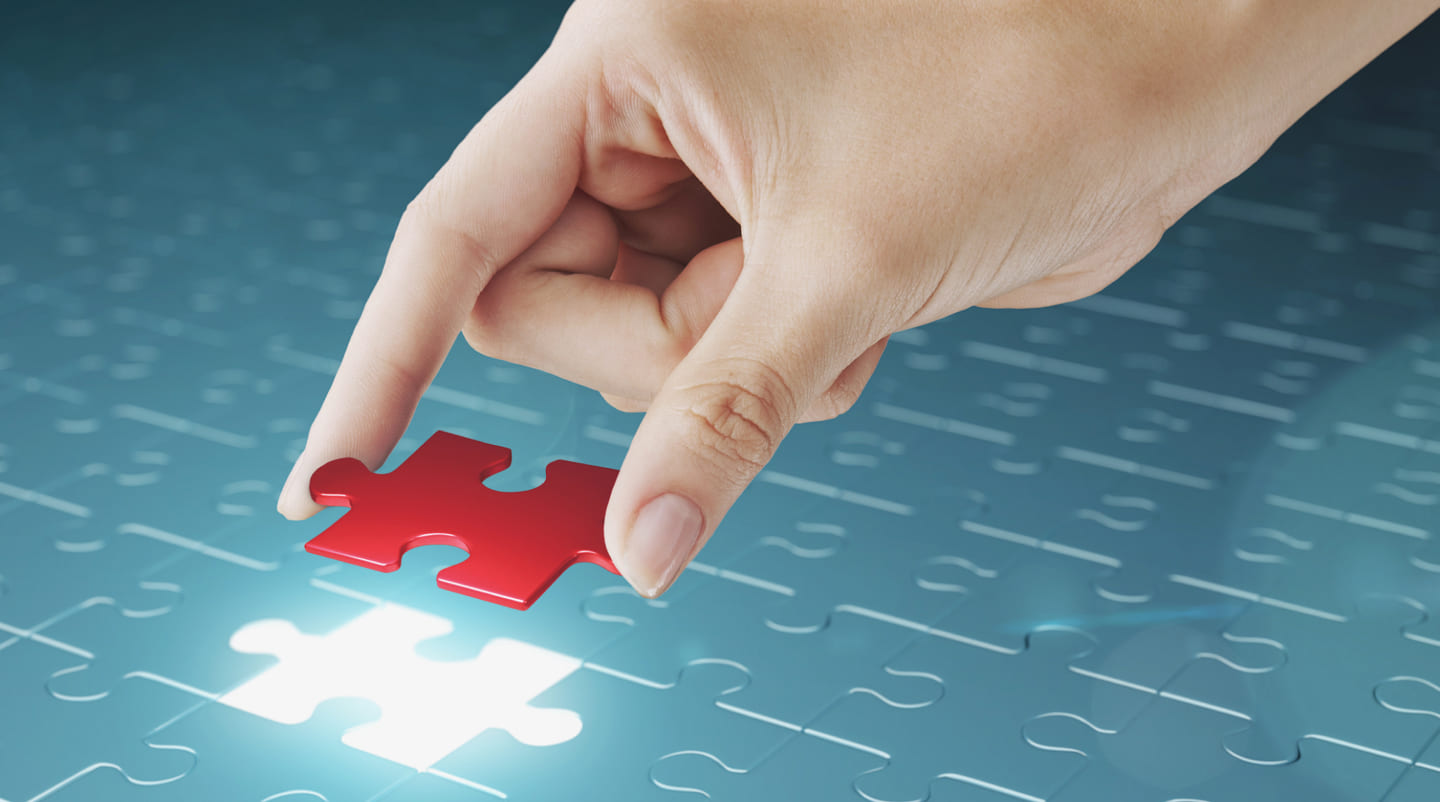
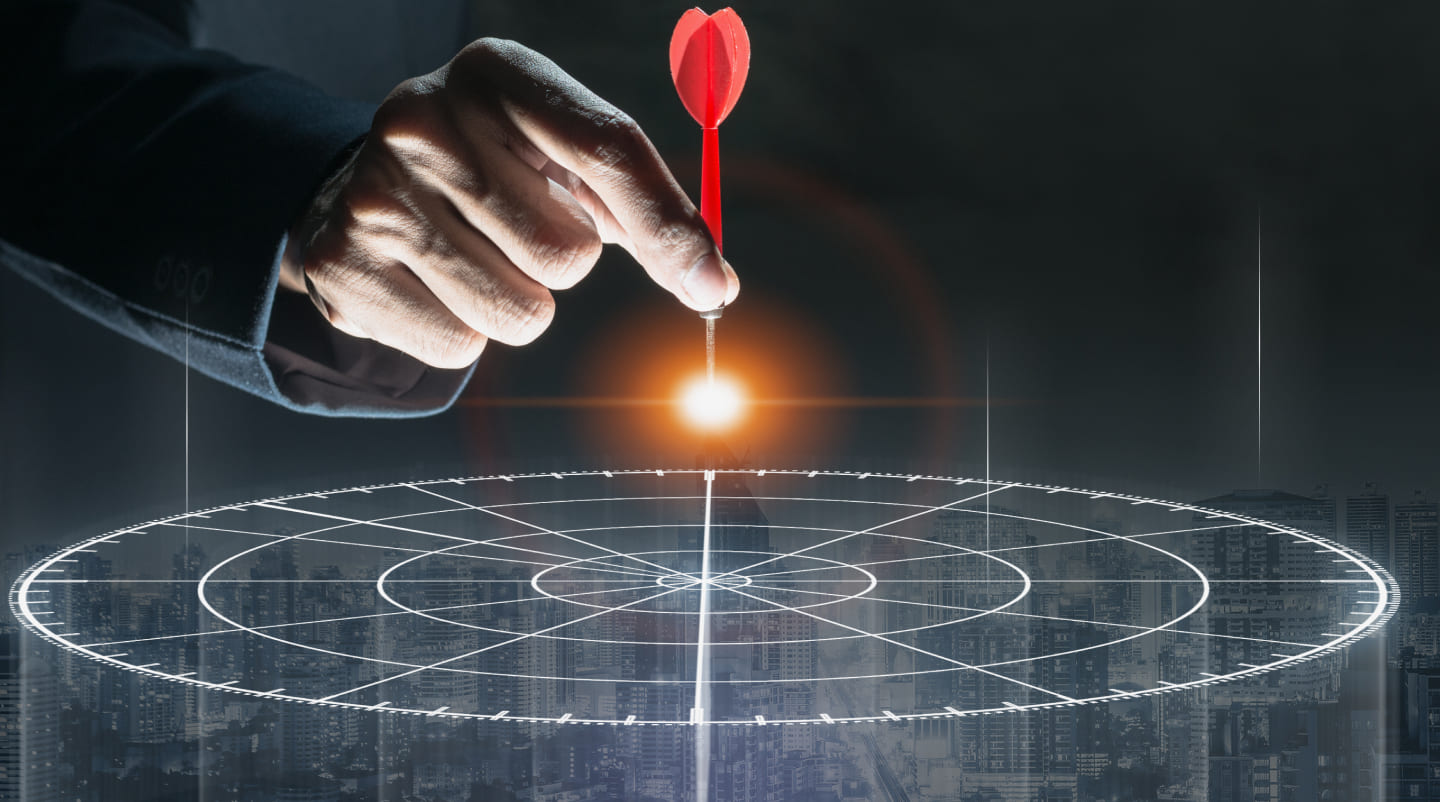
With Proprietary STF Composite Analysis Function, Achieving 98% Accuracy
- Ensuring 98% accuracy through composite analysis of vibration spectrum, trend analysis, and fault frequency
- Accurate diagnosis is achieved by integrating and analyzing various data, including those from diverse process environments
Benefits of Implementing ExRBM
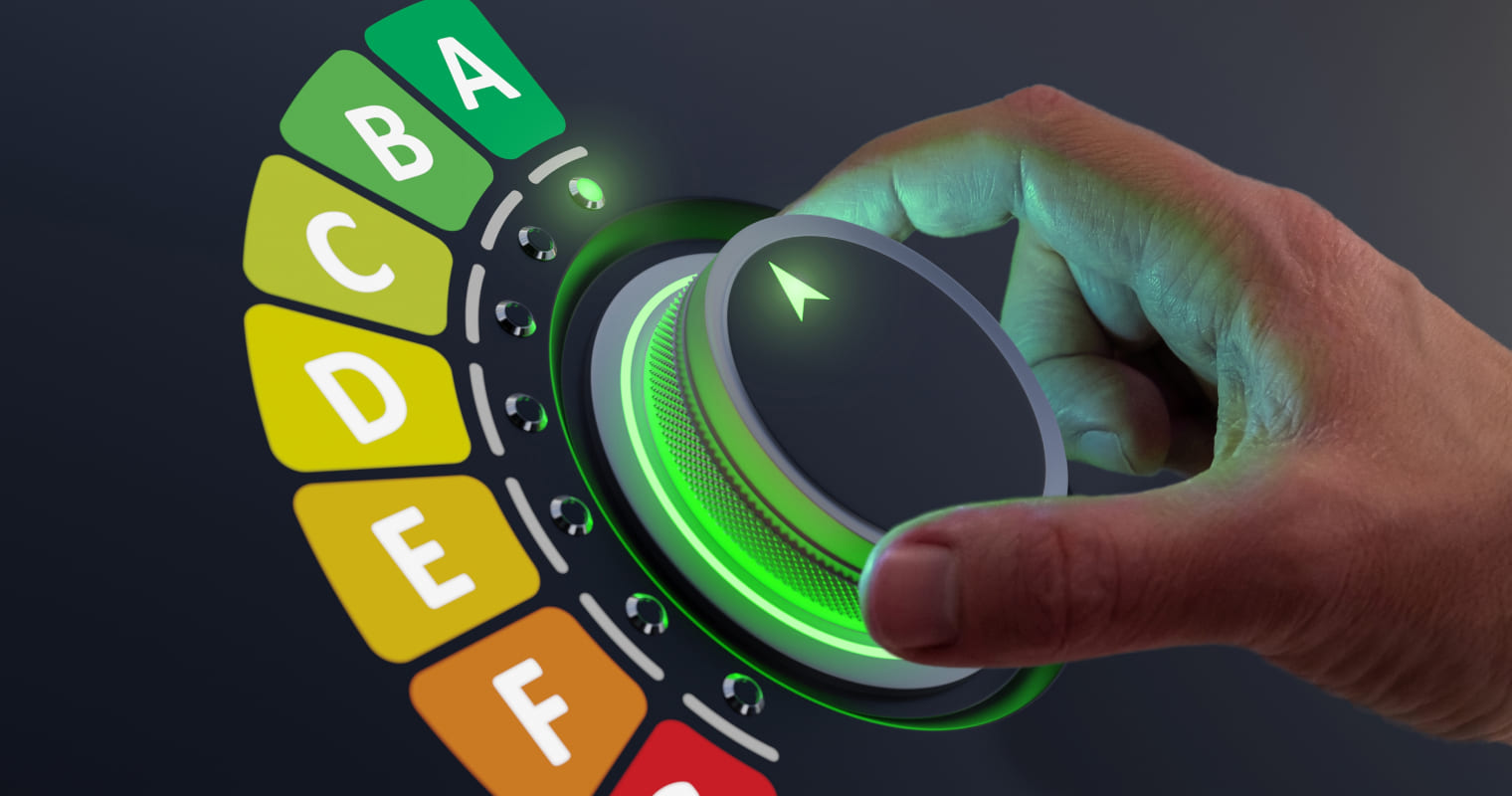
Improving Overall Operational Efficiency through Enhanced machinery Stability
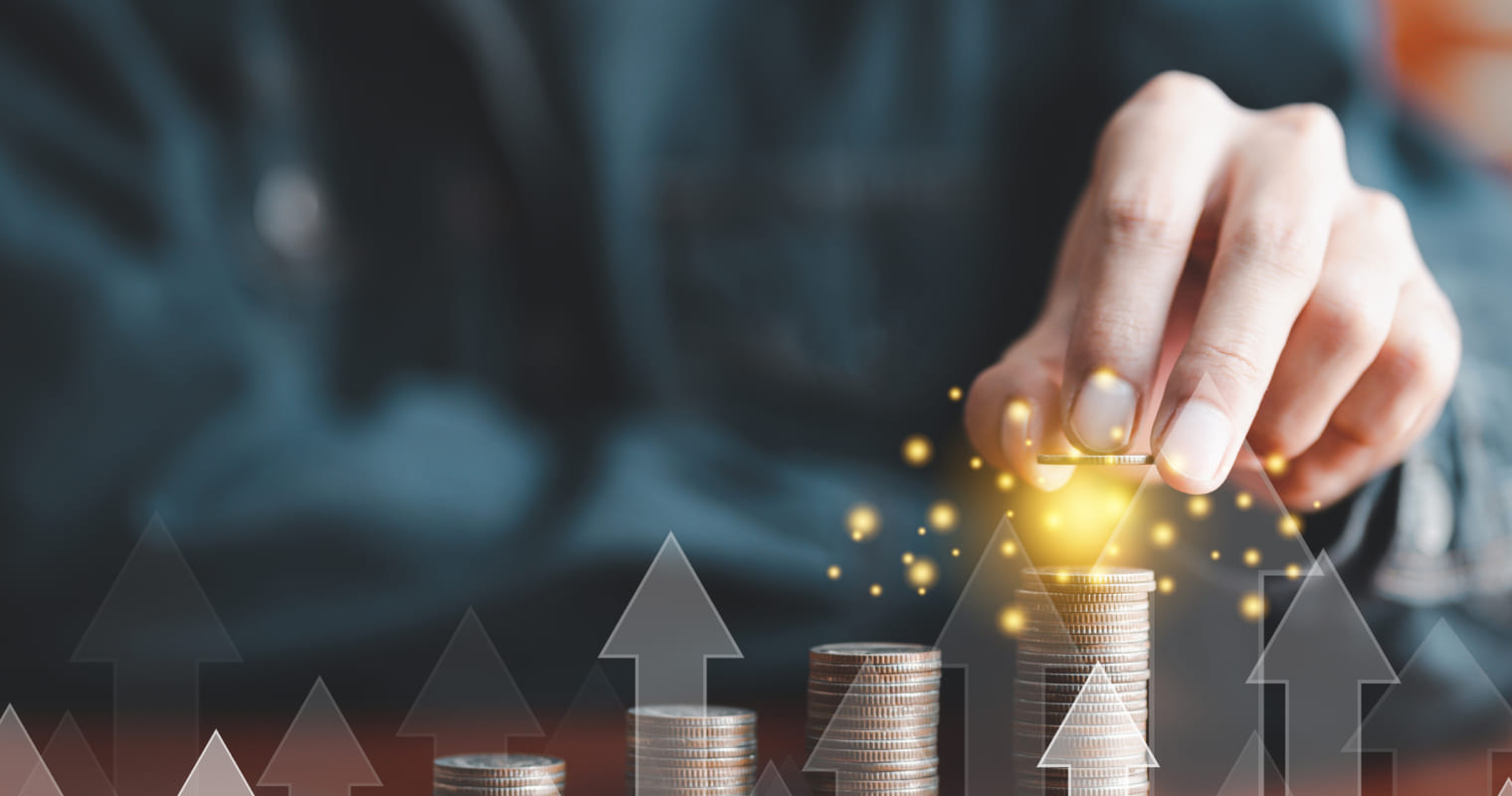
Enhanced Profitability through Cost Savings in Maintenance Expenses
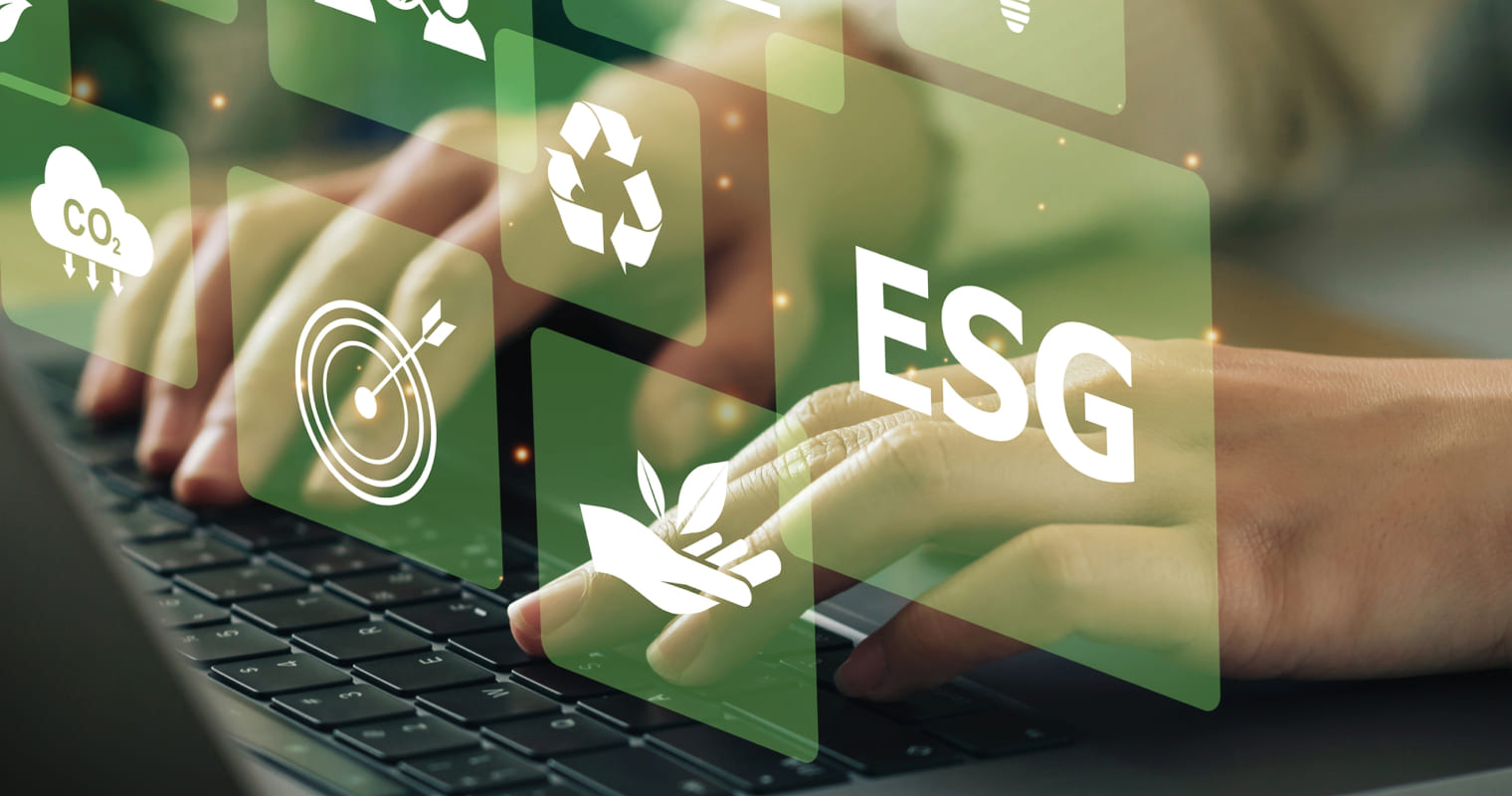
Efficient Implementation of ESG Management through the Establishment of Smart Factories
FAQ
The current method of diagnosis by experts involves measuring vibrations in the machinery and diagnosing faults based on the experience of the expert. Therefore, the same fault can be diagnosed differently by different experts, and even cases of completely different results and misdiagnoses are common. This can be compared to how doctors may diagnose patients differently.
At FutureMain, we take this issue very seriously and have developed a platform to address it. Firstly, we analyzed all types and mechanisms of faults occurring in machinery and classified each cause into 10 categories. We then designed an artificial intelligence (neural network theory) algorithm. As a result, we have ensured reliability to provide more accurate diagnoses than even expert diagnosticians.
Furthermore, in collaboration with the Korea Testing Laboratory (KTL), we applied ExRBM to operational machinery and conducted reliability assessments by diagnosing the machinery, proving an accuracy rate of 98.3%. We validated the reliability of ExRBM through more than 120 cases.
At FutureMain, we take this issue very seriously and have developed a platform to address it. Firstly, we analyzed all types and mechanisms of faults occurring in machinery and classified each cause into 10 categories. We then designed an artificial intelligence (neural network theory) algorithm. As a result, we have ensured reliability to provide more accurate diagnoses than even expert diagnosticians.
Furthermore, in collaboration with the Korea Testing Laboratory (KTL), we applied ExRBM to operational machinery and conducted reliability assessments by diagnosing the machinery, proving an accuracy rate of 98.3%. We validated the reliability of ExRBM through more than 120 cases.
In ExRBM, we analyze the fault mechanisms separately for machinery and its components and design diagnostic algorithms based on this analysis, enabling the diagnosis of machinery. The most commonly used single machinery in factories are rotational and reciprocating motion machinery, including motors, pumps, fans and blowers, centrifugal compressors, reciprocating compressors, and turbines, which are the primary targets of ExRBM. Even machinery with different purposes and functions, such as generators, which share a similar structure with motors, are subject to the same fault mechanisms.
Additionally, other factories consist of combinations of these machinery types. For instance, the Booster Feed Pump, comprised of a motor and a pump, can be diagnosed for overall faults by applying the respective fault mechanisms to each component. This versatility allows for the diagnosis of all machinery present in a factory. Moreover, algorithms analyzing fault mechanisms for each machinery component are integrated into the platform, enabling machinery diagnosis based on these algorithms. The platform covers components such as journal bearings, sliding bearings, simple gears, planetary gears, seals, couplings, belts, and others, making diagnosis possible for any component indicating faults. Furthermore, ExRBM includes a customization feature to add newly manufactured machinery to the platform for diagnosis, ensuring usability across any factory or process.
Additionally, other factories consist of combinations of these machinery types. For instance, the Booster Feed Pump, comprised of a motor and a pump, can be diagnosed for overall faults by applying the respective fault mechanisms to each component. This versatility allows for the diagnosis of all machinery present in a factory. Moreover, algorithms analyzing fault mechanisms for each machinery component are integrated into the platform, enabling machinery diagnosis based on these algorithms. The platform covers components such as journal bearings, sliding bearings, simple gears, planetary gears, seals, couplings, belts, and others, making diagnosis possible for any component indicating faults. Furthermore, ExRBM includes a customization feature to add newly manufactured machinery to the platform for diagnosis, ensuring usability across any factory or process.
Contact Us
Interested in FutureMain’s solutions?
Leave your request and we will get back to you promptly.
Leave your request and we will get back to you promptly.